RLAIF [Reinforcement Learning and Artificial Intelligence Framework] for Resource Management: Optimizing Energy Consumption
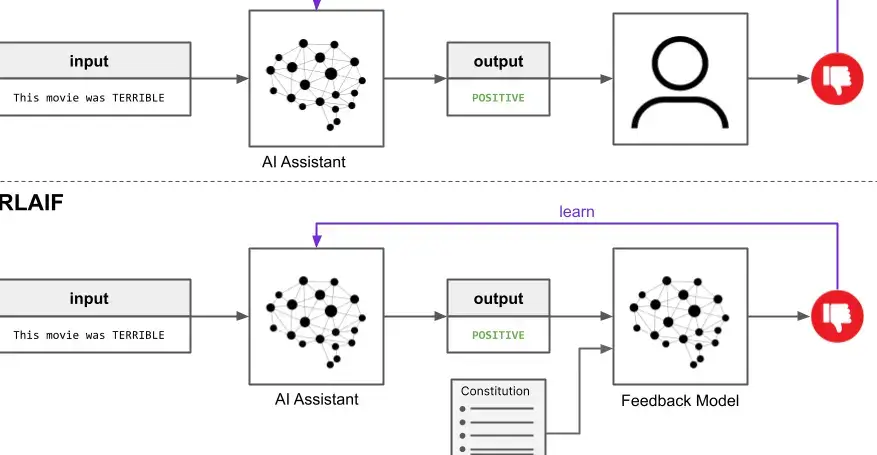
RLAIF
In today’s world, where sustainability and efficient resource management are of importance, Reinforcement Learning and Artificial intelligence Frameworks (RLAIF) have emerged as powerful tools, for optimizing energy usage. This article delves into how RLAIF can revolutionize resource management with a focus on energy consumption. By harnessing the capabilities of RLAIF we can pave the way for an environmentally conscious future.
Understanding RLAIF in Resource Management:
RLAIF combines techniques from reinforcement learning and artificial intelligence frameworks to create systems that learn from their surroundings and make decisions. When applied to resource management in the context of energy consumption RLAIF enables adaptive solutions that optimize energy usage minimize waste and reduce our ecological footprint.
Optimizing Energy Consumption:
RLAIF offers benefits when it comes to optimizing energy consumption. By analyzing data from sources like meters, weather patterns and occupancy levels RLAIF models can identify patterns and make intelligent decisions in real time. This allows for the discovery of opportunities to save energy by adjusting temperature settings optimizing lighting systems and managing high energy processes efficiently.
Dynamic Demand Response:
One significant application of RLAIF in managing energy consumption is dynamic demand response. Through modeling techniques within RLAIF models we can anticipate patterns, in energy demand. Adjust energy supply accordingly. By adjusting energy consumption to, off peak times or utilizing energy sources whenever possible RLAIF can assist in stabilizing the energy grid lessening stress during high demand periods and decreasing dependence, on fossil fuels.
Adaptive Energy Systems:
RLAIF can also play a role, in the advancement of energy systems. These systems are equipped with sensors and AI algorithms that constantly monitor energy usage and adjust accordingly to changing circumstances. By learning from data, user preferences and external factors RLAIF models optimize real time energy consumption to ensure resource utilization while maintaining comfort and productivity.
Addressing Energy Waste:
Another notable advantage of RLAIF in resource management is its capability to identify and address energy waste. Through analyzing energy consumption patterns RLAIF models can detect anomalies, equipment malfunctions or inefficient processes that result in energy usage. This enables interventions such as maintenance or process improvements leading to reduced energy waste and cost savings.
Challenges and Considerations:
While RLAIF offers potential for optimizing energy consumption it is crucial to address associated challenges and considerations. Privacy concerns, data security and algorithmic transparency must be carefully managed to build trust and acceptance, for RLAIF based solutions. Additionally continuous monitoring and updating of RLAIF models are essential to adapt to evolving technologies and changing energy patterns.
Conclusion:
RLAIF shows promise in revolutionizing resource management particularly when it comes to optimizing energy consumption. By harnessing the capabilities of RLAIF we can develop systems that adapt to varying circumstances optimize energy consumption and reduce waste. As we work towards a future solution based on RLAIF can play a crucial role in minimizing our environmental footprint while improving efficiency and saving costs. Embracing RLAIF, for resource management represents a stride, towards constructing an eco-friendlier and sustainable planet. Read more